By integrating AI, active learning, and automation, early-phase drug discovery is becoming faster, more efficient, and more targeted in the search for effective therapies.
Artificial intelligence is rapidly transforming the field of drug discovery, enabling researchers to navigate the immense complexity of chemical space with unprecedented precision and efficiency. German company Evotec is one of the global leaders in integrated drug discovery and development. Through advanced in-silico chemistry, Bayesian optimization, and machine learning models, Evotec focuses on identifying and optimizing active compounds, minimizing trial-and-error cycles, reducing synthesis workloads, and accelerating the path from target to therapy.
By leveraging active learning strategies and a robust automation infrastructure, Evotec supports a shift from traditional high-throughput methods to data-driven, iterative screening, where AI models prioritize the most informative compounds for testing. This allows researchers to achieve superior outcomes with fewer experimental resources, enabling the discovery of novel chemical series and improved therapeutic candidates with a significantly higher probability of clinical success.
Navigating Vast Chemical Space with AI-Driven Precision
One of the greatest challenges in modern drug discovery is the sheer scale of chemical space.
“When we search for active molecules, we’re navigating an astronomical space,” explained Lionel Colliandre, In-Silico Chemistry expert at Evotec, during his presentation at Forum Labo in Paris last March.
“The space of small drug-like molecules is estimated at 10⁶⁰ possibilities, and if we’re looking at therapeutic proteins, we’re dealing with a space of 10³⁹⁰. These are numbers far beyond what any traditional approach can handle.”
In such a vast landscape, the ability to pinpoint viable drug candidates requires more than brute force—it demands intelligent prioritization.
This is where Evotec’s application of AI and machine learning becomes essential. By integrating predictive models and high-quality experimental data, the company tackles multi-objective optimization, where activity, solubility, toxicity, and developability must all be considered simultaneously.
“The real challenge isn’t just finding active compounds—it’s optimizing them across multiple parameters with a limited number of synthesized candidates,” Colliandre noted.
Through this approach, Evotec minimizes experimental overhead while maintaining a high standard of compound viability, fundamentally shifting how early-phase medicinal chemistry is conducted.
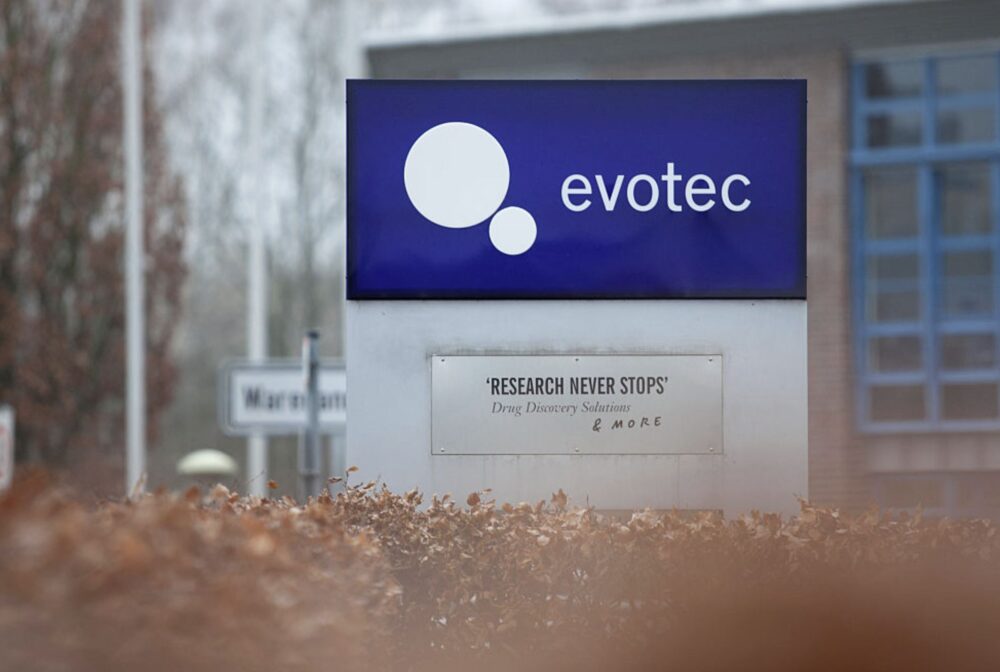
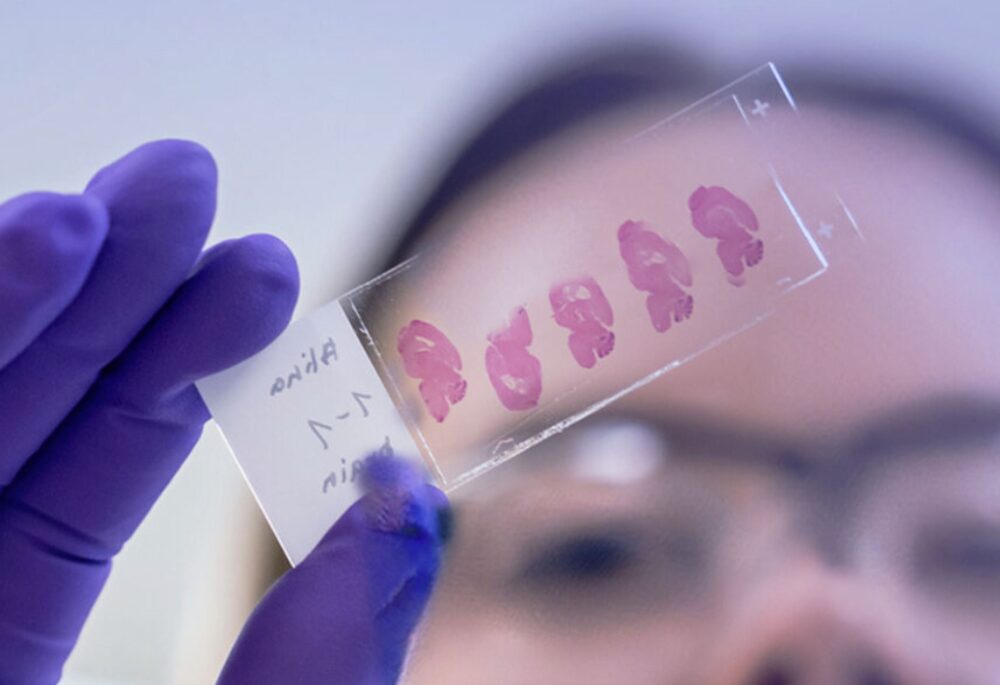
Smarter Screening Through Bayesian Optimization and Iterative Learning
To overcome the inefficiencies of traditional screening methods, Evotec employs Bayesian optimization, a machine learning technique that intelligently guides experimental decisions.
“The goal is to find the maximum of a function—in this case, the best compound—using the fewest possible iterations,” said Colliandre. “Each experimental test is chosen based on the expected information gain for the next step.”
This method balances exploration of uncertain chemical regions with exploitation of known high-potential areas, enabling researchers to uncover optimal compounds faster and more efficiently than random or exhaustive screening.
Evotec enhances this process with iterative machine learning cycles that adapt based on real-time data. Starting from a small initial dataset, models are trained to predict the most promising candidates from a vast library. These predictions are tested in small batches, and the results are fed back into the system for the next round.
“With this approach, we were able to identify 80% of active compounds by screening just 20% of the library,” Colliandre shared.
Furthermore, AI-driven molecular design tools—ranging from generative models to docking simulations—contribute to each optimization cycle.
“Whether the molecules come from a medicinal chemist’s brain or a generative algorithm, what matters is that we can rank and select them based on data. It’s not just faster—it’s smarter.”
Automation and Active Learning: Accelerating the Path to Therapies
A key enabler of Evotec’s AI-powered discovery engine is its robust automation infrastructure. From high-throughput compound screening to sample management and plate preparation, the company has built an ecosystem where robotics and machine learning operate in tandem.
“Automation is critical,” emphasized Colliandre. “It allows us to run rapid, iterative experiments—sometimes in batches of just a few thousand compounds—without compromising accuracy or speed.”
This integration ensures that active learning cycles can scale effectively, even in scenarios where traditional high-throughput screening is impractical due to assay time constraints or cost.
Ultimately, this approach aligns with Evotec’s broader mission to bring “medicines that matter” to patients more efficiently. By uniting automation, AI, and cross-functional expertise, the company achieves a data-driven, modular workflow that’s both adaptable and cost-effective.
“We’re not just optimizing molecules—we’re optimizing the entire discovery process,” Colliandre concluded.
This methodology doesn’t just reduce development time and resource consumption; it increases the likelihood of clinical success, supporting Evotec’s role as a forward-thinking partner in the co-creation of next-generation therapies.